Tang uses deep learning to infer 3D world from 2D videos
Tang uses deep learning to infer 3D world from 2D videos
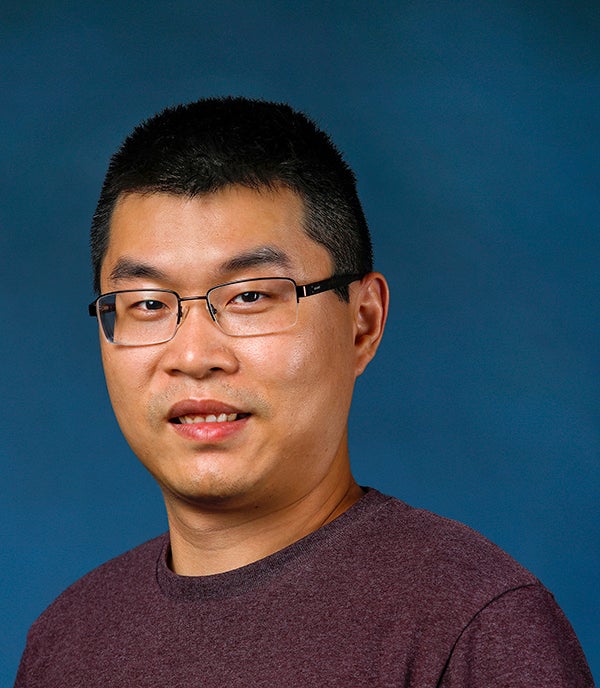
Assistant Professor Wei Tang is developing circuits and systems that can use two-dimensional images to navigate the real, three-dimensional world in real-time.
Deep learning, a branch of machine learning, has drastically advanced vision-based environment perception. It builds an artificial neural network to “learn” to recognize objects, faces, and all manners of data. Just as a human brain learns by repetition, computers are trained on multiple data sets, so their accuracy improves. Deep neural networks are composed of multiple layers stacked on top of each other, designed to refine and improve results.
Deep learning methods depend on 3D annotation, a process that labels data to make it usable for the models. This tedious process includes mapping where various elements are within a space using cuboids and ensuring the model understands the relationships between different objects in that space. This is critical for cyber-physical systems such as robots used in either a manufacturing or personal setting, or in autonomous vehicles. Also, this work must be conducted in a controlled environment, making translating it to everyday applications difficult, if not impossible.
By using streaming videos, Tang will be able to train the system using readily available 2D annotations to not only recognize objects and scene layouts but to estimate their 3D geometry and 3D motion in real-time. He aims to establish a self-supervised framework that lifts 2D objects to 3D, scaling them to perceive their entire environment.
“The outcomes of this project will facilitate a wide range of applications, from robots in manufacturing and personal services to autonomous vehicles that enhance people’s mobility and safety,” Tang said. “Furthermore, this project will tightly integrate research and education at UIC, which is a Minority Serving Institution through curriculum development, research training for high school, undergraduate, and graduate students, broadening the participation of female and minority students, and community outreach.”
Tang received a three-year, $240,000 grant from the National Science Foundation (NSF), Modeling and Learning Space-time Structures for Real-time Environment Perception, in support of this project. This is his first NSF grant.