Ziebart receives AAAI Classic Paper Award
Ziebart receives AAAI Classic Paper Award
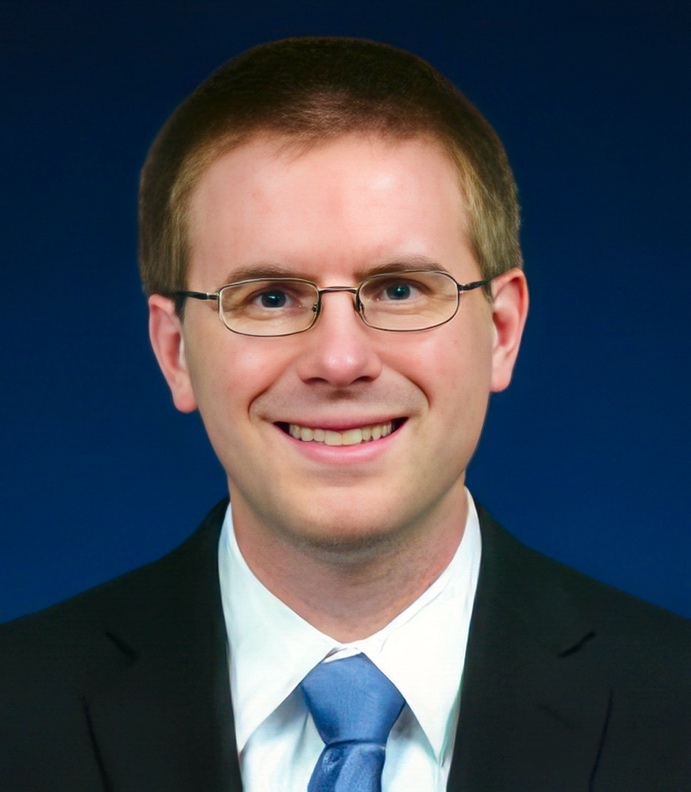
Associate Professor Brian Ziebart received the 2024 Association for the Advancement of Artificial Intelligence Classic Paper Award. The award honors the authors of papers deemed most influential, chosen from a specific conference year.
Ziebart, along with Andrew Maas, J. Andrew Bagnell, and Anind K. Dey authored the paper “Maximum Entropy Inverse Reinforcement Learning” in 2008. The paper has been cited 3,300 times since its publication and was acknowledged for introducing entropy regularization to reinforcement learning that led to improvements in the predictive accuracy of forecasting, imitation learning, decision making, and human-AI alignment.
AI models are trained to predict the behavior and decisions that would be chosen by a human, such as which particular route a driver would take home from work. Sequential decision-making behavior can be difficult for machine learning algorithms.
When the paper was published 16 years ago, learning a reward function that produces optimal behavior in a Markov decision process was a new idea for approaching problems of imitation learning.
A Markov decision process provides a mathematical framework for modeling decision making in situations that are partly random and partly under the control of a decision maker. They are traditionally used to obtain the decisions producing behavior that maximizes a known reward function. The noisiness of human behavior makes learning the reward function that produces demonstrated behavior not just difficult, but ill-posed. Maximum Entropy Inverse Reinforcement Learning resolves this issue by finding the most uncertain distribution over decision policies that is guaranteed to match the reward of demonstrated behavior on average.
Ziebart and his co-author’s research was motivated by the real-world routing preference of drivers. The team applied their approach to route preference modeling using 100,000 miles of GPS data of taxicab drivers. Knowing the road network, speed limits, number of lanes, and actions available, the group was able to extend route preferences with hidden goals to infer future routes and destinations.
Their novel approach to inverse reinforcement and imitation learning resolved ambiguities in previous approaches to behavior prediction. It also provided important connections between decision theory and information theory that have been widely adopted as “entropy regularization” in reinforcement learning methods used to train large language models like ChatGPT.