Health Data Science Consortium explores NLP applications
Focus on cross-campus collaboration
Health Data Science Consortium holds first meeting: Heading link
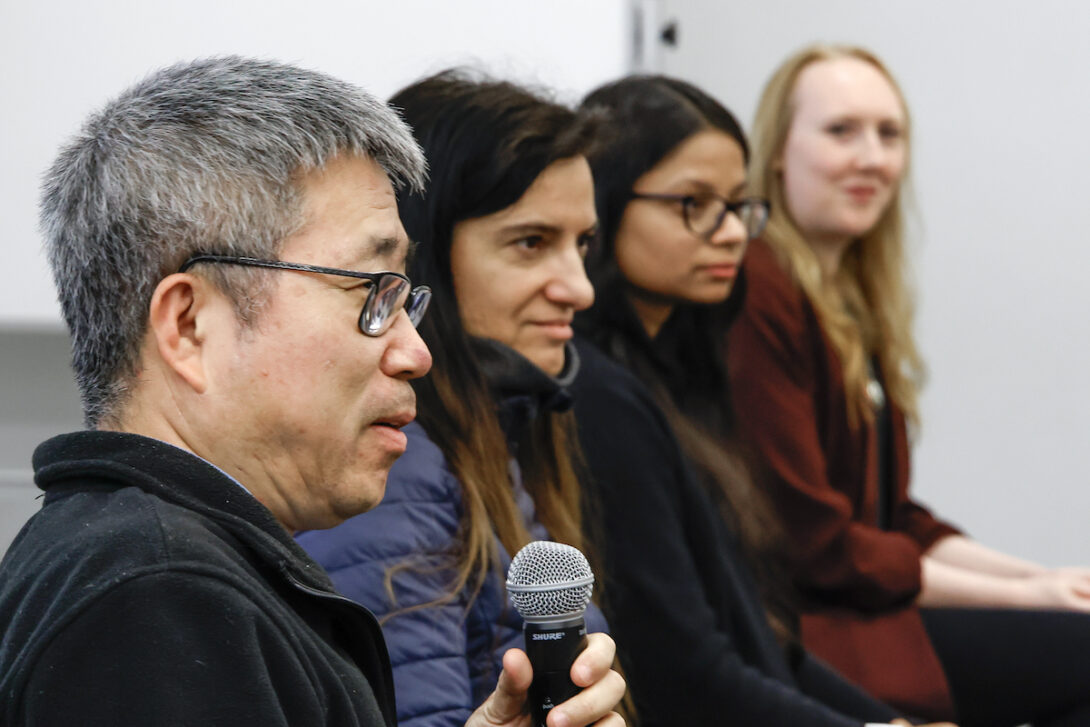
UIC’s Center for Clinical and Translational Science, which helps UIC researchers bring health breakthroughs into the world faster, held its second Health Data Science Consortium meeting on January 22. The session, “NLP: From Theoretical Research to Health Sciences Applications,” featured four UIC computer science professors who work in Natural Language Processing (NLP). By the end of the meeting, several researchers from both the health sciences and computer science were discussing opportunities to collaborate.
The field of NLP, a subset of AI, is focused on enabling computers to understand and generate human language. NLP is what allows conversational agents such as Amazon’s Alexa or Apple’s Siri to listen to queries and find answers, chatbots to function, and Chat-GPT to generate prose.
Distinguished Professor Bing Liu, Professor Cornelia Caragea, Assistant Professor Natalie Parde, and Assistant Professor Shweta Yadav described the conceptual framework of NLP, and the health science applications of the technology being investigated at UIC.
Liu’s work in sentiment analysis systems infers people’s opinions from text, especially on social media. This includes parsing online reviews, and determining whether they are authentic or fake. He has improved sentiment analysis tools with machine lifelong learning, algorithms that can transfer past knowledge to a current task to improve accuracy. These tools can be used to examine reviews of doctors or hospitals or suss out the type of information people with a certain health condition may be seeking online.
Caragea, who is currently serving as a program director for the National Science Foundation, conducts research in NLP, AI, deep learning, and information retrieval. Deep learning models can supply wrong answers and can be overconfident in the accuracy of the answers they provide – something that would have minimal detrimental effect if someone is seeking the name of a band’s third album, but could be harmful relating to healthcare matters. She is training models by feeding algorithms both hard and easy questions, which has resulted in better-calibrated models. When confidence in an answer isn’t at a certain threshold, the question can be routed to a higher-level, more accurate model, or a human, to ensure accuracy.
Parde’s work is at the intersection of NLP and healthcare, focusing primarily on aspects of cognition, health behavior, and caregiver support. She is developing ways to automatically detect linguistic and verbal features of psychotic disorders, to improve automated mental health support and screening tools. She also is working to improve early childhood interventions for infants and toddlers from racially and ethnically diverse and socially disadvantaged families.
Before joining UIC, Yadav was a postdoctoral research fellow at the U.S. National Library of Medicine at the National Institutes of Health. Her research interests lie at the intersection of NLP, healthcare informatics, biomedical text mining, and computational social science. She highlighted the enormous amount of healthcare-related data generated from electronic medical records, wearable mobile devices, and social media posts, which account for about 30 percent of the world’s data volume and noted that each day in the U.S. over eight million searches for medical information are performed.
Yadav is developing a robust question-answering system explicitly designed to meet the information needs of healthcare consumers. This includes providing faster responses to consumers’ healthcare questions by offering them reliable and trustworthy answers and providing a rationale for why these results were deemed most accurate. She is also designing a practical decision support system that sifts through users’ social media posts and can provide healthcare professionals with deeper insights into users’ depressive behaviors by capturing fine-grained depressive symptoms.
The presentations were followed by a Q&A, and questions ranged among those related to the methods employed to process data with NLP, such as the accuracy of models, ways to identify missing data, how to compensate for overconfidence in a particular model, how models are created to identify which data are important, and HIPAA implications of using AI on patient data (the panelists assured the questioner that it is always stripped of identifying information before use). Other questions were more theoretical in nature, on how to move AI from the lab to the real world, and various potential uses of AI in healthcare.
Additional Health Data Science Consortium seminars will be held this semester; check the Center for Clinical and Translational Science website to learn more.